Variational Fourier Features in the GPflow framework#
In this notebook we demonstrate how new types of inducing variables can easily be incorporated in the GPflow framework. As an example case, we use the variational Fourier features from Hensman, Durrande, and Solin (JMLR 2018). All equation and table references are to this paper.
Note: This implementation is meant as an example, not as a feature-complete implementation. For more features, such as multi-dimensional inputs, use the GPflow 2 version of the original VFF code.
We cannot directly use Fourier features within the multi-output framework without losing the computational advantages, as Kuu
and Kuf
for SharedIndependent and SeparateIndependent inducing variables assume that the sub-inducing variable’s covariances are simply computed as dense Tensors. However, there is nothing preventing a dedicated implementation of multi-output Fourier features that is computationally more efficient - feel free to discuss this within the GPflow
community!
[1]:
import tensorflow as tf
import numpy as np
import gpflow
from gpflow.inducing_variables import InducingVariables
from gpflow.base import TensorLike
from gpflow.utilities import to_default_float
from gpflow import covariances as cov
from gpflow import kullback_leiblers as kl
from gpflow.ci_utils import reduce_in_tests
from gpflow.experimental.check_shapes import Shape
2022-09-20 14:29:09.207124: I tensorflow/core/platform/cpu_feature_guard.cc:193] This TensorFlow binary is optimized with oneAPI Deep Neural Network Library (oneDNN) to use the following CPU instructions in performance-critical operations: AVX2 AVX512F FMA
To enable them in other operations, rebuild TensorFlow with the appropriate compiler flags.
2022-09-20 14:29:09.353236: W tensorflow/stream_executor/platform/default/dso_loader.cc:64] Could not load dynamic library 'libcudart.so.11.0'; dlerror: libcudart.so.11.0: cannot open shared object file: No such file or directory
2022-09-20 14:29:09.353259: I tensorflow/stream_executor/cuda/cudart_stub.cc:29] Ignore above cudart dlerror if you do not have a GPU set up on your machine.
2022-09-20 14:29:09.382369: E tensorflow/stream_executor/cuda/cuda_blas.cc:2981] Unable to register cuBLAS factory: Attempting to register factory for plugin cuBLAS when one has already been registered
2022-09-20 14:29:10.194647: W tensorflow/stream_executor/platform/default/dso_loader.cc:64] Could not load dynamic library 'libnvinfer.so.7'; dlerror: libnvinfer.so.7: cannot open shared object file: No such file or directory
2022-09-20 14:29:10.194714: W tensorflow/stream_executor/platform/default/dso_loader.cc:64] Could not load dynamic library 'libnvinfer_plugin.so.7'; dlerror: libnvinfer_plugin.so.7: cannot open shared object file: No such file or directory
2022-09-20 14:29:10.194722: W tensorflow/compiler/tf2tensorrt/utils/py_utils.cc:38] TF-TRT Warning: Cannot dlopen some TensorRT libraries. If you would like to use Nvidia GPU with TensorRT, please make sure the missing libraries mentioned above are installed properly.
/home/circleci/project/gpflow/experimental/utils.py:42: UserWarning: You're calling gpflow.experimental.check_shapes.decorator.check_shapes which is considered *experimental*. Expect: breaking changes, poor documentation, and bugs.
warn(
/home/circleci/project/gpflow/experimental/utils.py:42: UserWarning: You're calling gpflow.experimental.check_shapes.inheritance.inherit_check_shapes which is considered *experimental*. Expect: breaking changes, poor documentation, and bugs.
warn(
[2]:
# VFF give structured covariance matrices that are computationally efficient.
# We take advantage of this using TensorFlow's LinearOperators:
BlockDiag = tf.linalg.LinearOperatorBlockDiag
Diag = tf.linalg.LinearOperatorDiag
LowRank = tf.linalg.LinearOperatorLowRankUpdate
[3]:
import matplotlib.pyplot as plt
%matplotlib inline
The VFF inducing variables are defined as a projection \(u_m = \mathcal{P}_{\phi_m}(f)\) (eq. (59)) of the GP \(f(\cdot)\) onto a truncated Fourier basis, \(\phi_m = [1, \cos(\omega_1(x-a)),\dots,\cos(\omega_M(x-a)),\sin(\omega_1(x-a)),\dots,\sin(\omega_M(x-a))]\) (eq. (47)). To represent this we define a new inducing variables class that derives from the InducingVariables
base class.
[4]:
class FourierFeatures1D(InducingVariables):
def __init__(self, a, b, M):
"""
`a` and `b` define the interval [a, b] of the Fourier representation.
`M` specifies the number of frequencies to use.
"""
# [a, b] defining the interval of the Fourier representation:
self.a = gpflow.Parameter(a, dtype=gpflow.default_float())
self.b = gpflow.Parameter(b, dtype=gpflow.default_float())
self.M = M
# integer array defining the frequencies, ω_m = 2π (b - a)/m:
self.ms = np.arange(M)
@property
def num_inducing(self):
""" number of inducing variables (defines dimensionality of q(u)) """
return 2 * self.M - 1 # `M` cosine and `M-1` sine components
@property
def shape(self) -> Shape:
M = 2 * self.M - 1
D = 1 # Input size.
P = 1 # Output size.
return (M, D, P)
Next, we need to define how to compute \(\mathrm{K}_\mathbf{uu} = \operatorname{cov}(u_m, u_{m'})\) (eq. (61)) and \(\mathrm{K}_\mathbf{uf} = \operatorname{cov}(u_m, f(x_n))\) (eq. (60)).
[5]:
@cov.Kuu.register(FourierFeatures1D, gpflow.kernels.Matern12)
def Kuu_matern12_fourierfeatures1d(inducing_variable, kernel, jitter=None):
a, b, ms = (lambda u: (u.a, u.b, u.ms))(inducing_variable)
omegas = 2.0 * np.pi * ms / (b - a)
# Cosine block:
lamb = 1.0 / kernel.lengthscales
two_or_four = to_default_float(tf.where(omegas == 0, 2.0, 4.0))
d_cos = (
(b - a) * (tf.square(lamb) + tf.square(omegas)) / lamb / kernel.variance / two_or_four
) # eq. (111)
v_cos = tf.ones_like(d_cos) / tf.sqrt(kernel.variance) # eq. (110)
cosine_block = LowRank(Diag(d_cos, is_positive_definite=True), v_cos[:, None])
# Sine block:
omegas = omegas[tf.not_equal(omegas, 0)] # the sine block does not include omega=0
d_sin = (
(b - a) * (tf.square(lamb) + tf.square(omegas)) / lamb / kernel.variance / 4.0
) # eq. (113)
sine_block = Diag(d_sin, is_positive_definite=True)
return BlockDiag([cosine_block, sine_block])
@cov.Kuf.register(FourierFeatures1D, gpflow.kernels.Matern12, TensorLike)
def Kuf_matern12_fourierfeatures1d(inducing_variable, kernel, X):
X = tf.squeeze(X, axis=1)
a, b, ms = (lambda u: (u.a, u.b, u.ms))(inducing_variable)
omegas = 2.0 * np.pi * ms / (b - a)
Kuf_cos = tf.cos(omegas[:, None] * (X[None, :] - a))
omegas_sin = omegas[tf.not_equal(omegas, 0)] # don't compute zero frequency
Kuf_sin = tf.sin(omegas_sin[:, None] * (X[None, :] - a))
# correct Kuf outside [a, b] -- see Table 1
Kuf_sin = tf.where((X < a) | (X > b), tf.zeros_like(Kuf_sin), Kuf_sin) # just zero
left_tail = tf.exp(-tf.abs(X - a) / kernel.lengthscales)[None, :]
right_tail = tf.exp(-tf.abs(X - b) / kernel.lengthscales)[None, :]
Kuf_cos = tf.where(X < a, left_tail, Kuf_cos) # replace with left tail
Kuf_cos = tf.where(X > b, right_tail, Kuf_cos) # replace with right tail
return tf.concat([Kuf_cos, Kuf_sin], axis=0)
@cov.Kuu.register(FourierFeatures1D, gpflow.kernels.Matern32)
def Kuu_matern32_fourierfeatures1d(inducing_variable, kernel, jitter=None):
a, b, ms = (lambda u: (u.a, u.b, u.ms))(inducing_variable)
omegas = 2.0 * np.pi * ms / (b - a)
# Cosine block: eq. (114)
lamb = np.sqrt(3.0) / kernel.lengthscales
four_or_eight = to_default_float(tf.where(omegas == 0, 4.0, 8.0))
d_cos = (
(b - a)
* tf.square(tf.square(lamb) + tf.square(omegas))
/ tf.pow(lamb, 3)
/ kernel.variance
/ four_or_eight
)
v_cos = tf.ones_like(d_cos) / tf.sqrt(kernel.variance)
cosine_block = LowRank(Diag(d_cos, is_positive_definite=True), v_cos[:, None])
# Sine block: eq. (115)
omegas = omegas[tf.not_equal(omegas, 0)] # don't compute omega=0
d_sin = (
(b - a)
* tf.square(tf.square(lamb) + tf.square(omegas))
/ tf.pow(lamb, 3)
/ kernel.variance
/ 8.0
)
v_sin = omegas / lamb / tf.sqrt(kernel.variance)
sine_block = LowRank(Diag(d_sin, is_positive_definite=True), v_sin[:, None])
return BlockDiag([cosine_block, sine_block]) # eq. (116)
@cov.Kuf.register(FourierFeatures1D, gpflow.kernels.Matern32, TensorLike)
def Kuf_matern32_fourierfeatures1d(inducing_variable, kernel, X):
X = tf.squeeze(X, axis=1)
a, b, ms = (lambda u: (u.a, u.b, u.ms))(inducing_variable)
omegas = 2.0 * np.pi * ms / (b - a)
Kuf_cos = tf.cos(omegas[:, None] * (X[None, :] - a))
omegas_sin = omegas[tf.not_equal(omegas, 0)] # don't compute zeros freq.
Kuf_sin = tf.sin(omegas_sin[:, None] * (X[None, :] - a))
# correct Kuf outside [a, b] -- see Table 1
def tail_cos(delta_X):
arg = np.sqrt(3) * tf.abs(delta_X) / kernel.lengthscales
return (1 + arg) * tf.exp(-arg)[None, :]
Kuf_cos = tf.where(X < a, tail_cos(X - a), Kuf_cos)
Kuf_cos = tf.where(X > b, tail_cos(X - b), Kuf_cos)
def tail_sin(delta_X):
arg = np.sqrt(3) * tf.abs(delta_X) / kernel.lengthscales
return delta_X[None, :] * tf.exp(-arg) * omegas_sin[:, None]
Kuf_sin = tf.where(X < a, tail_sin(X - a), Kuf_sin)
Kuf_sin = tf.where(X > b, tail_sin(X - b), Kuf_sin)
return tf.concat([Kuf_cos, Kuf_sin], axis=0)
In principle, this is all we need; however, to be able to take advantage of the structure of Kuu
, we need to also implement new versions of the KL divergence from the prior to the approximate posterior (prior_kl
) and the computation of the Gaussian process conditional (posterior) equations:
[6]:
@kl.prior_kl.register(FourierFeatures1D, gpflow.kernels.Kernel, TensorLike, TensorLike)
def prior_kl_vff(inducing_variable, kernel, q_mu, q_sqrt, whiten=False):
if whiten:
raise NotImplementedError
K = cov.Kuu(inducing_variable, kernel)
return gauss_kl_vff(q_mu, q_sqrt, K)
def gauss_kl_vff(q_mu, q_sqrt, K):
"""
Compute the KL divergence from
q(x) = N(q_mu, q_sqrt^2)
to
p(x) = N(0, K)
q_mu is a vector [N, 1] that contains the mean.
q_sqrt is a matrix that is the lower triangular square-root matrix of the covariance of q.
K is a positive definite matrix: the covariance of p.
NOTE: K is a LinearOperator that provides efficient methjods
for solve(), log_abs_determinant(), and trace()
"""
# KL(N₀ || N₁) = ½ [tr(Σ₁⁻¹ Σ₀) + (μ₁ - μ₀)ᵀ Σ₁⁻¹ (μ₁ - μ₀) - k + ln(det(Σ₁)/det(Σ₀))]
# N₀ = q; μ₀ = q_mu, Σ₀ = q_sqrt q_sqrtᵀ
# N₁ = p; μ₁ = 0, Σ₁ = K
# KL(q || p) =
# ½ [tr(K⁻¹ q_sqrt q_sqrtᵀA + q_muᵀ K⁻¹ q_mu - k + logdet(K) - logdet(q_sqrt q_sqrtᵀ)]
# k = number of dimensions, if q_sqrt is m x m this is m²
Kinv_q_mu = K.solve(q_mu)
mahalanobis_term = tf.squeeze(tf.matmul(q_mu, Kinv_q_mu, transpose_a=True))
# GPflow: q_sqrt is num_latent_gps x N x N
num_latent_gps = to_default_float(tf.shape(q_mu)[1])
logdet_prior = num_latent_gps * K.log_abs_determinant()
product_of_dimensions__int = tf.reduce_prod(tf.shape(q_sqrt)[:-1]) # dimensions are integers
constant_term = to_default_float(product_of_dimensions__int)
Lq = tf.linalg.band_part(q_sqrt, -1, 0) # force lower triangle
logdet_q = tf.reduce_sum(tf.math.log(tf.square(tf.linalg.diag_part(Lq))))
# S = tf.matmul(q_sqrt, q_sqrt, transpose_b=True)
# trace_term = tf.trace(K.solve(S))
trace_term = tf.squeeze(
tf.reduce_sum(Lq * K.solve(Lq), axis=[-1, -2])
) # [O(N²) instead of O(N³)
twoKL = trace_term + mahalanobis_term - constant_term + logdet_prior - logdet_q
return 0.5 * twoKL
[7]:
import gpflow.posteriors
class VFFPosterior(gpflow.posteriors.BasePosterior):
def _conditional_fused(self, Xnew, full_cov, full_output_cov):
"""
Xnew is a tensor with the points of the data or minibatch, shape N x D
"""
if full_output_cov:
raise NotImplementedError
f = self._q_dist.q_mu
q_sqrt = self._q_dist.q_sqrt
# num_data = tf.shape(Xnew)[0] # M
num_func = tf.shape(f)[1] # K
Kuu = cov.Kuu(self.X_data, self.kernel) # this is now a LinearOperator
Kuf = cov.Kuf(self.X_data, self.kernel, Xnew) # still a Tensor
KuuInv_Kuf = Kuu.solve(Kuf)
# compute the covariance due to the conditioning
if full_cov:
fvar = self.kernel(Xnew) - tf.matmul(Kuf, KuuInv_Kuf, transpose_a=True)
shape = (num_func, 1, 1)
else:
KufT_KuuInv_Kuf_diag = tf.reduce_sum(Kuf * KuuInv_Kuf, axis=-2)
fvar = self.kernel(Xnew, full_cov=False) - KufT_KuuInv_Kuf_diag
shape = (num_func, 1)
fvar = tf.expand_dims(fvar, 0) * tf.ones(
shape, dtype=gpflow.default_float()
) # K x N x N or K x N
if self.whiten:
raise NotImplementedError
A = KuuInv_Kuf
# construct the conditional mean
fmean = tf.matmul(A, f, transpose_a=True)
if q_sqrt is not None:
if q_sqrt.get_shape().ndims == 2:
# LTA = A * tf.expand_dims(q_sqrt, 2) # K x M x N
# won't work # make ticket for this?
raise NotImplementedError
elif q_sqrt.get_shape().ndims == 3:
# L = tf.matrix_band_part(tf.transpose(q_sqrt, (2, 0, 1)), -1, 0) # K x M x M
# K x M x N
# A_tiled = tf.expand_dims(A.get(), 0) * tf.ones((num_func, 1, 1), dtype=float_type)
# LTA = tf.matmul(L, A_tiled, transpose_a=True) # K x M x N
# TODO the following won't work for K > 1
assert q_sqrt.shape[0] == 1
# LTA = (A.T @ DenseMatrix(q_sqrt[:,:,0])).T.get()[None, :, :]
ATL = tf.matmul(A, q_sqrt, transpose_a=True)
else:
raise ValueError("Bad dimension for q_sqrt: %s" % str(q_sqrt.get_shape().ndims))
if full_cov:
# fvar = fvar + tf.matmul(LTA, LTA, transpose_a=True) # K x N x N
fvar = fvar + tf.matmul(ATL, ATL, transpose_b=True) # K x N x N
else:
# fvar = fvar + tf.reduce_sum(tf.square(LTA), 1) # K x N
fvar = fvar + tf.reduce_sum(tf.square(ATL), 2) # K x N
if not full_cov:
fvar = tf.transpose(fvar) # N x K
return fmean, fvar
# We can also provide a conditional that precomputes as much as possible,
# to speed up predictions:
def _precompute(self):
Kuu = cov.Kuu(self.X_data, self.kernel) # this is now a LinearOperator
q_mu = self._q_dist.q_mu
q_sqrt = self._q_dist.q_sqrt
if self.whiten:
raise NotImplementedError
else:
# alpha = Kuu⁻¹ q_mu
alpha = Kuu.solve(q_mu) # type: tf.Tensor
if self.whiten:
raise NotImplementedError
else:
# Qinv = Kuu⁻¹ - Kuu⁻¹ S Kuu⁻¹
KuuInv_qsqrt = Kuu.solve(q_sqrt)
KuuInv_covu_KuuInv = tf.matmul(KuuInv_qsqrt, KuuInv_qsqrt, transpose_b=True)
Qinv = Kuu.inverse().to_dense() - KuuInv_covu_KuuInv
return gpflow.posteriors.PrecomputedValue.wrap_alpha_Qinv(alpha, Qinv)
def _conditional_with_precompute(self, cache, Xnew, full_cov, full_output_cov):
alpha, Qinv = cache
if full_output_cov:
raise NotImplementedError
Kuf = cov.Kuf(self.X_data, self.kernel, Xnew) # still a Tensor
# construct the conditional mean
fmean = tf.matmul(Kuf, alpha, transpose_a=True)
num_func = tf.shape(alpha)[1] # K
Qinv_Kuf = tf.matmul(Qinv, Kuf)
# compute the covariance due to the conditioning
if full_cov:
fvar = self.kernel(Xnew) - tf.matmul(Kuf, Qinv_Kuf, transpose_a=True)
else:
KufT_Qinv_Kuf_diag = tf.reduce_sum(Kuf * Qinv_Kuf, axis=-2)
fvar = self.kernel(Xnew, full_cov=False) - KufT_Qinv_Kuf_diag
fvar = tf.transpose(fvar)
return fmean, fvar
We now have to register our Posterior object:
[8]:
@gpflow.posteriors.get_posterior_class.register(gpflow.kernels.Kernel, FourierFeatures1D)
def _get_posterior_vff(kernel, inducing_variable):
return VFFPosterior
gpflow.conditionals.conditional
is a short-hand for calling the fused prediction code path:
[9]:
Mf = 2
M = 2 * Mf - 1
kernel = gpflow.kernels.Matern32()
inducing_variable = FourierFeatures1D(-0.5, 1.5, Mf)
Xnew = np.random.rand(7, 1)
f = np.random.randn(M, 1)
q_sqrt = tf.convert_to_tensor(np.tril(np.random.randn(1, M, M)))
conditional_f_mean, conditional_f_var = gpflow.conditionals.conditional(
Xnew, inducing_variable, kernel, f, q_sqrt=q_sqrt, white=False, full_cov=True
)
posterior = VFFPosterior(kernel, inducing_variable, f, q_sqrt, whiten=False, precompute_cache=None)
posterior_f_mean, posterior_f_var = posterior.fused_predict_f(Xnew, full_cov=True)
np.testing.assert_array_equal(conditional_f_mean, posterior_f_mean)
np.testing.assert_array_equal(conditional_f_var, posterior_f_var)
/home/circleci/project/gpflow/experimental/utils.py:42: UserWarning: You're calling gpflow.experimental.check_shapes.checker.ShapeChecker.__init__ which is considered *experimental*. Expect: breaking changes, poor documentation, and bugs.
warn(
2022-09-20 14:29:13.341308: W tensorflow/stream_executor/platform/default/dso_loader.cc:64] Could not load dynamic library 'libcuda.so.1'; dlerror: libcuda.so.1: cannot open shared object file: No such file or directory
2022-09-20 14:29:13.341336: W tensorflow/stream_executor/cuda/cuda_driver.cc:263] failed call to cuInit: UNKNOWN ERROR (303)
2022-09-20 14:29:13.341356: I tensorflow/stream_executor/cuda/cuda_diagnostics.cc:156] kernel driver does not appear to be running on this host (947c84c595a3): /proc/driver/nvidia/version does not exist
2022-09-20 14:29:13.341603: I tensorflow/core/platform/cpu_feature_guard.cc:193] This TensorFlow binary is optimized with oneAPI Deep Neural Network Library (oneDNN) to use the following CPU instructions in performance-critical operations: AVX2 AVX512F FMA
To enable them in other operations, rebuild TensorFlow with the appropriate compiler flags.
We can also call the cached path:
[10]:
posterior.update_cache(gpflow.posteriors.PrecomputeCacheType.TENSOR)
precomputed_posterior_f_mean, precomputed_posterior_f_var = posterior.predict_f(Xnew, full_cov=True)
np.testing.assert_allclose(precomputed_posterior_f_mean, posterior_f_mean)
np.testing.assert_allclose(precomputed_posterior_f_var, posterior_f_var)
We now demonstrate how to use these new types of inducing variables with the SVGP
model class. First, let’s create some toy data:
[11]:
X = np.linspace(-2, 2, 510)
Xnew = np.linspace(-4, 4, 501)
def f(x):
return np.cos(2 * np.pi * x / 4 * 2)
F = f(X)
Fnew = f(Xnew)
noise_scale = 0.1
np.random.seed(1)
Y = F + np.random.randn(*F.shape) * noise_scale
data = (X.reshape(-1, 1), Y.reshape(-1, 1))
[12]:
plt.figure()
plt.plot(X, F, label="f(x)")
plt.plot(X, Y, ".", label="observations")
plt.legend()
plt.show()
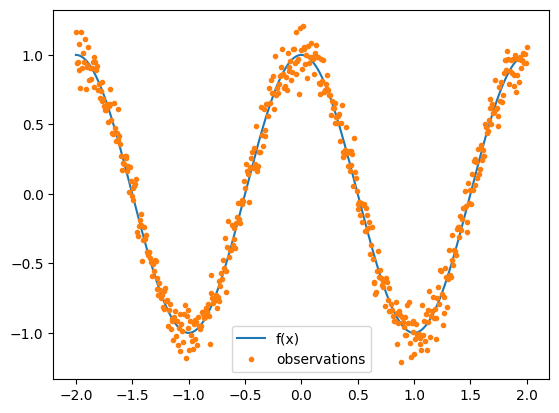
Setting up an SVGP model with variational Fourier feature inducing variables is as simple as replacing the inducing_variable
argument:
[13]:
Mfreq = 9
m = gpflow.models.SVGP(
kernel=gpflow.kernels.Matern32(),
likelihood=gpflow.likelihoods.Gaussian(variance=noise_scale ** 2),
inducing_variable=FourierFeatures1D(-4.5, 4.5, Mfreq),
num_data=len(X),
whiten=False,
)
gpflow.set_trainable(m.kernel, False)
gpflow.set_trainable(m.likelihood, False)
gpflow.set_trainable(m.inducing_variable, True) # whether to optimize bounds [a, b]
[14]:
opt = gpflow.optimizers.Scipy()
opt.minimize(
m.training_loss_closure(data),
m.trainable_variables,
options=dict(maxiter=reduce_in_tests(5000)),
)
gpflow.utilities.print_summary(m, fmt="notebook")
name | class | transform | prior | trainable | shape | dtype | value |
---|---|---|---|---|---|---|---|
SVGP.kernel.variance | Parameter | Softplus | False | () | float64 | 1.0 | |
SVGP.kernel.lengthscales | Parameter | Softplus | False | () | float64 | 1.0 | |
SVGP.likelihood.variance | Parameter | Softplus + Shift | False | () | float64 | 0.01 | |
SVGP.inducing_variable.a | Parameter | Identity | True | () | float64 | -4.18351 | |
SVGP.inducing_variable.b | Parameter | Identity | True | () | float64 | 4.18402 | |
SVGP.q_mu | Parameter | Identity | True | (17, 1) | float64 | [[0.89075... | |
SVGP.q_sqrt | Parameter | FillTriangular | True | (1, 17, 17) | float64 | [[[1.35057e+00, 0.00000e+00, 0.00000e+00... |
For comparison we also construct an SVGP model using inducing points and an exact GPR model:
[15]:
m_ip = gpflow.models.SVGP(
kernel=gpflow.kernels.Matern32(),
likelihood=gpflow.likelihoods.Gaussian(variance=noise_scale ** 2),
inducing_variable=np.linspace(-2, 2, Mfreq * 2 - 1)[:, None],
num_data=len(X),
whiten=False,
)
gpflow.set_trainable(m_ip.kernel, False)
gpflow.set_trainable(m_ip.likelihood, False)
gpflow.set_trainable(m_ip.inducing_variable, True) # whether to optimize inducing point locations
[16]:
opt = gpflow.optimizers.Scipy()
opt.minimize(
m_ip.training_loss_closure(data),
m_ip.trainable_variables,
options=dict(maxiter=reduce_in_tests(5000)),
)
gpflow.utilities.print_summary(m_ip, fmt="notebook")
name | class | transform | prior | trainable | shape | dtype | value |
---|---|---|---|---|---|---|---|
SVGP.kernel.variance | Parameter | Softplus | False | () | float64 | 1.0 | |
SVGP.kernel.lengthscales | Parameter | Softplus | False | () | float64 | 1.0 | |
SVGP.likelihood.variance | Parameter | Softplus + Shift | False | () | float64 | 0.01 | |
SVGP.inducing_variable.Z | Parameter | Identity | True | (17, 1) | float64 | [[-1.93002... | |
SVGP.q_mu | Parameter | Identity | True | (17, 1) | float64 | [[0.96123... | |
SVGP.q_sqrt | Parameter | FillTriangular | True | (1, 17, 17) | float64 | [[[2.165e-02, 0.000e+00, 0.000e+00... |
[17]:
m_ref = gpflow.models.GPR((X.reshape(-1, 1), Y.reshape(-1, 1)), kernel=gpflow.kernels.Matern32())
m_ref.likelihood.variance = np.array(noise_scale ** 2).astype(np.float64)
gpflow.set_trainable(m_ref.kernel, False)
gpflow.set_trainable(m_ref.likelihood, False)
# Because we fixed the kernel and likelihood hyperparameters, we don't need to optimize anything.
gpflow.utilities.print_summary(m_ref, fmt="notebook")
name | class | transform | prior | trainable | shape | dtype | value |
---|---|---|---|---|---|---|---|
GPR.kernel.variance | Parameter | Softplus | False | () | float64 | 1 | |
GPR.kernel.lengthscales | Parameter | Softplus | False | () | float64 | 1 |
[18]:
exact_gpr_lml = m_ref.log_marginal_likelihood().numpy().item()
print("LML (exact GPR) =", exact_gpr_lml)
ip_svgp_elbo = m_ip.elbo(data).numpy().item()
print("ELBO (SVGP, inducing points) =", ip_svgp_elbo)
vff_svgp_elbo = m.elbo(data).numpy().item()
print("ELBO (SVGP, Fourier features) =", vff_svgp_elbo)
LML (exact GPR) = 402.2158074486284
ELBO (SVGP, inducing points) = 365.31215466596103
ELBO (SVGP, Fourier features) = 171.03923686986113
[19]:
def plot_gp(m, Xnew, name=""):
Fmean, Fvar = m.predict_f(Xnew[:, None])
Fmean = Fmean.numpy().squeeze()
Fvar = Fvar.numpy().squeeze()
(p,) = plt.plot(Xnew, Fmean, label=name)
plt.fill_between(
Xnew, Fmean - 2 * np.sqrt(Fvar), Fmean + 2 * np.sqrt(Fvar), alpha=0.3, color=p.get_color()
)
def plot_data():
plt.plot(Xnew, Fnew, label="f(x)")
plt.plot(X, Y, ".", label="observations")
plt.figure(figsize=(15, 10))
plot_data()
plot_gp(m, Xnew, "VFF [ELBO={:.3}]".format(vff_svgp_elbo))
plot_gp(m_ip, Xnew, "inducing points [ELBO={:.3}]".format(ip_svgp_elbo))
plot_gp(m_ref, Xnew, "exact [LML={:.3}]".format(exact_gpr_lml))
plt.legend(loc="best")
plt.show()
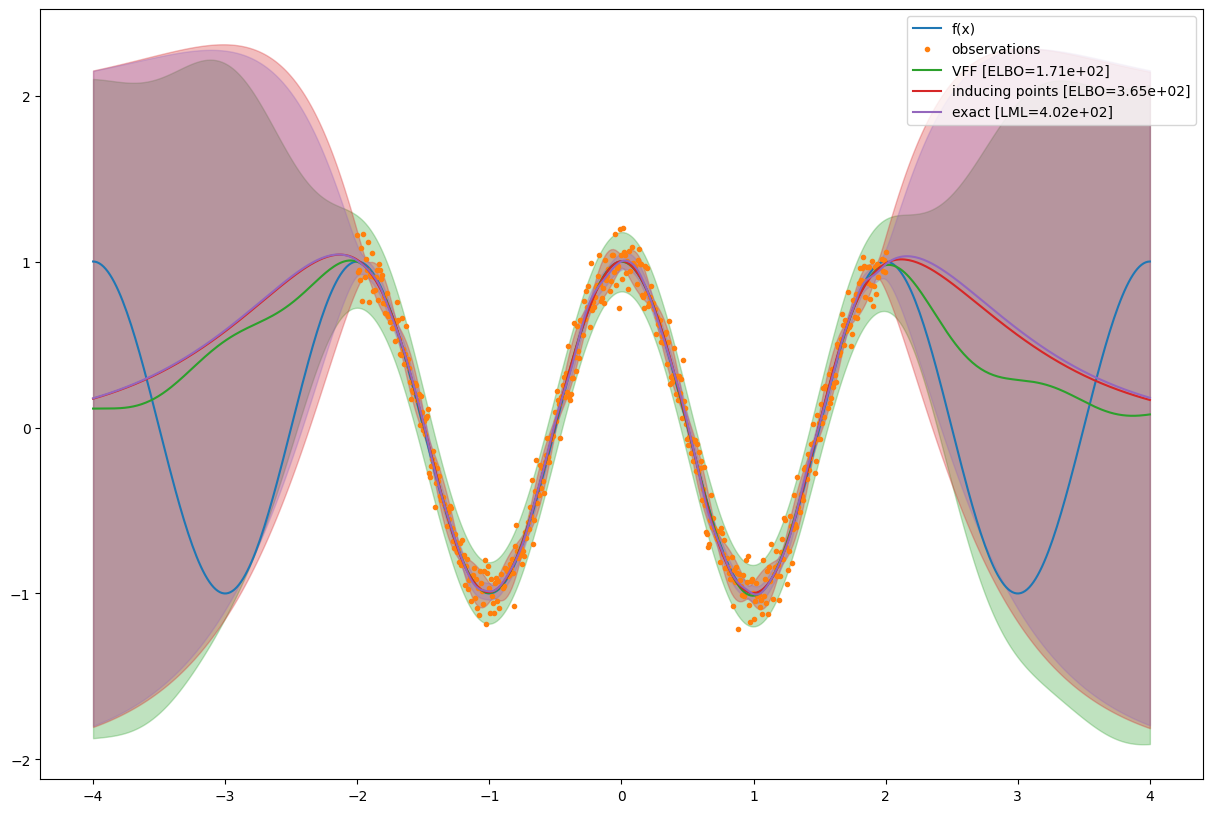